Bold, Confident, and Timeless: Introducing SKIMS x Dolce & Gabbana
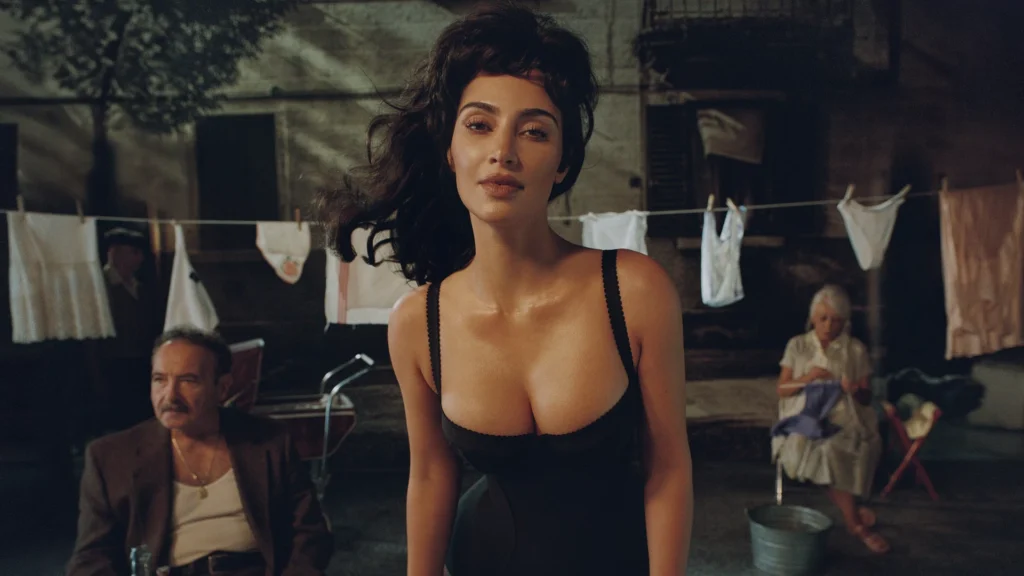
Kim Kardashian’s shapewear label, SKIMS, has unveiled an exciting collaboration with the iconic Italian luxury brand Dolce & Gabbana. This limited-edition collection, launching on November 19, 2024, promises to merge the sophistication of Italian design with the unparalleled comfort and functionality of SKIMS shapewear. The SKIMS x Dolce & Gabbana collection is a celebration of the female silhouette, embodying sensuality, confidence, and effortlessness. By combining Dolce & Gabbana’s print-forward, couture-inspired aesthetics with SKIMS’ expertise in comfort and fit, this collaboration creates a wardrobe designed to inspire and empower. With the holiday season approaching, the collection arrives just in time for Thanksgiving and Christmas shoppers looking for the perfect gift or indulgent treat. From silk lingerie to sculpting bodysuits, these pieces elevate everyday wear with bold yet refined touches. Kim Kardashian offered fans a glimpse of the collaboration through her Instagram, showcasing an array of luxurious pieces: Classic Two-Piece Sets in pristine white. Leopard Print Bodysuits that exude boldness and flair. Sheer Black Mesh Corsets paired with over-the-knee stockings. Sculpting Leggings and Solid Black Bodysuits for a sleek silhouette. Statement D&G accents, including scarves, bandanas, and jewelry. Each item is adorned with Dolce & Gabbana’s signature elegance and SKIMS’ iconic logo, creating a distinct look that effortlessly transitions from day to night. Shop at Skims > Shop at Dolce & Gabbana >
Donald Trump Taps Dr. Oz to Head U.S. Medicaid & Medicare
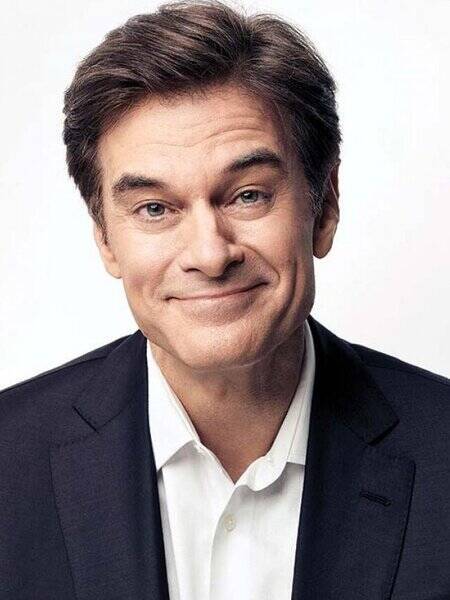
President-elect Donald Trump has nominated Dr. Mehmet Oz, a well-known television personality and surgeon, to lead the Centers for Medicare and Medicaid Services (CMS). Oz, who will oversee programs impacting millions of Americans, is known for his media presence and health advocacy. However, some have criticized his promotion of treatments lacking scientific support. Trump’s selection of Oz highlights his unconventional approach to leadership, blending celebrity influence with healthcare reform goals. Related Podcast Trump Taps Dr. Oz for Medicaid & Medicare President-elect Donald Trump has nominated Dr. Mehmet Oz, a well-known television personality and surgeon, to lead the Centers for Medicare and Medicaid Services (CMS).